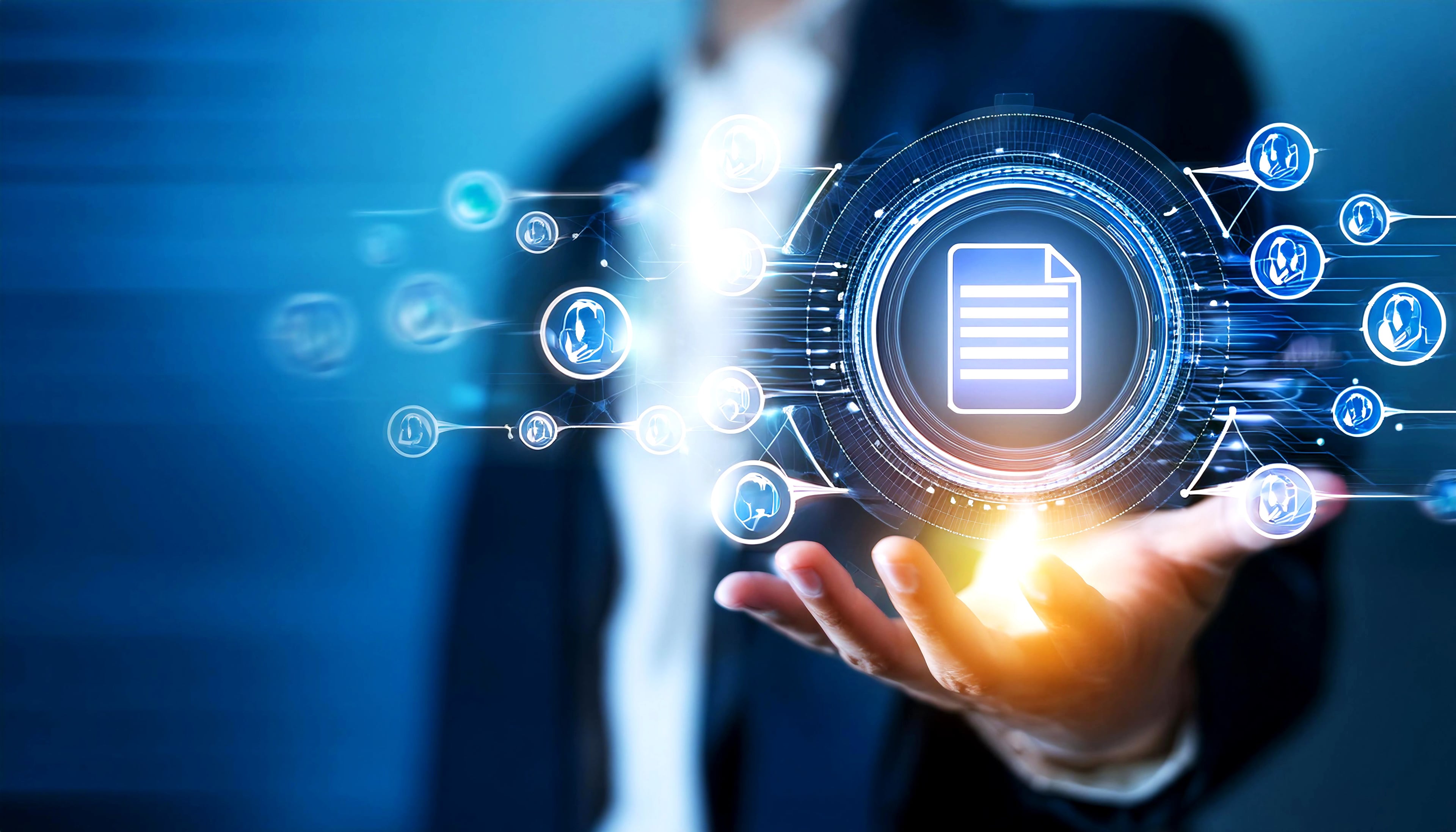
Mastering the Data Governance Framework: Best Practices and Key Benefits
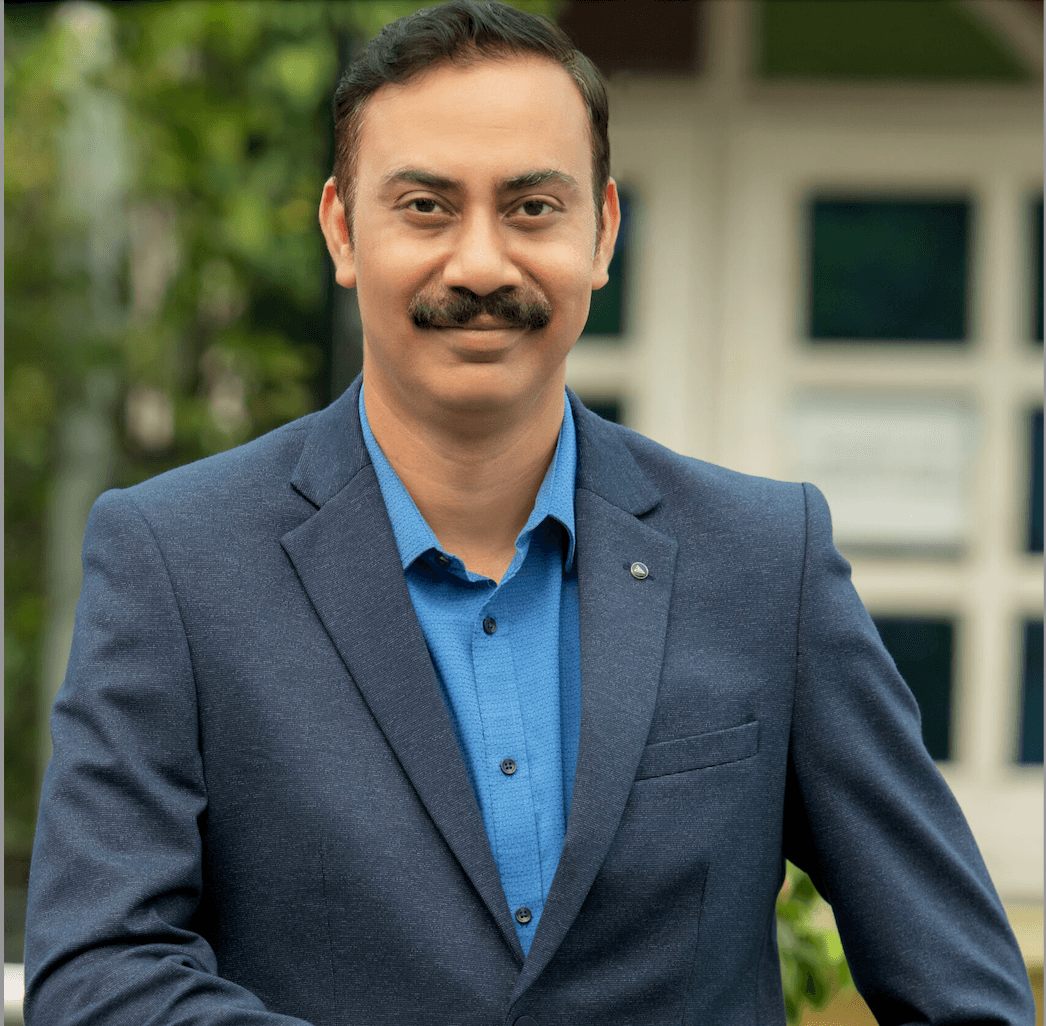
Key Takeaways
- A data governance framework is essential for ensuring data quality, regulatory compliance, and effective decision-making within organizations.
- Key components of a successful data governance framework include clearly defined policies, established roles, and advanced metadata management to enhance data discoverability and governance.
- Implementing performance metrics and fostering a culture of data literacy are critical for continuous improvement and overcoming challenges in data governance initiatives.
Understanding the Data Governance Framework

A data governance framework refers to more than just a set of guidelines—it’s the backbone of your organization’s data strategy and compliance efforts. It provides a structured approach for overseeing data management processes, ensuring data quality, and maintaining regulatory compliance.
Establishing clear policies and procedures within a robust data governance framework ensures data integrity and secure management, granting appropriate access to data when needed.
Importance of Data Governance Frameworks
A data governance framework fosters trust in data, crucial for effective decision-making. Mitigating risks associated with data misuse and maintaining data integrity allows organizations to leverage their data more effectively. Real-world success stories illustrate significant improvements in data accuracy and regulatory compliance following the implementation of structured data governance frameworks.
Moreover, effective metadata management enhances user understanding of data by providing essential context regarding data origins, meanings, and uses.
Key Components of a Data Governance Framework
A well-structured data governance framework includes ten essential components:
- Policies and rules
- Processes and organizational structures
- Technologies
- Mission statements
- Goals
- Success measurement
- Decision-making responsibilities
Clear governance policies for data access define who can access sensitive data and track misuse, ensuring data security. Technology plays a critical role in maintaining the security, integrity, lineage, usability, and availability of data within the governance framework.
Building a Data Governance Strategy

Developing a data governance strategy follows next. Typically, a Chief Data Officer (CDO), an executive sponsor, or a dedicated data governance manager leads a successful program.
This strategy involves setting clear goals and objectives, identifying data owners and stewards, and developing comprehensive data governance policies.
Setting Clear Goals and Objectives
Specific objectives are vital for measuring the success of data governance and aligning with business needs. Evaluating the effectiveness of data governance involves using measurable metrics and key performance indicators. The rate of data usage indicates how effectively teams are utilizing data assets within the organization.
Effective stakeholder engagement and a well-structured framework are essential for successful data governance.
Identifying Data Owners and Stewards
Dedicated roles like data stewards, responsible for enforcing data policies, are crucial. Effective data governance also needs accountable roles and a champion to lead change. The Chief Data Officer (CDO) plays a pivotal role in leading data governance initiatives and establishing a data governance structure.
Responsibilities of data stewards include managing datasets, enforcing rules, and handling day-to-day data needs.
Developing Data Governance Policies
Comprehensive governance policies outline data access protocols, ensuring clarity on handling sensitive data. A comprehensive metadata repository aids in governance by maintaining consistent data definitions and standards across the organization.
Rapid data growth necessitates additional governance strategies to manage new types of data effectively.
Implementing Data Governance Processes
Implementing data governance processes involves several critical elements, including data creation, management, and protection processes. Formal processes ensure consistent execution and enforcement of policies.
Information steward applications help with data profiling. They also monitor the performance of governance policies.
Data Mapping and Classification
Data mapping involves understanding where data resides and how data flows within an organization. Classifying datasets considers privacy or sensitivity issues, organizing information by importance and sensitivity.
Effective metadata management enhances data discovery by providing context about data content and structure.
Ensuring Data Quality and Integrity
Data quality scores assess the accuracy, consistency, and reliability of data, serving as key performance metrics. Periodic audits and quality control checks of data validity and procedural compliance are necessary.
Integrating data catalogs into data governance strategies helps maintain data quality and compliance by tracking data lineage and usage.
Compliance with Regulatory Requirements
Adherence to data privacy regulations like GDPR and HIPAA improves compliance and protects organizational reputation. Regular audits are essential to ensure data security and compliance with relevant regulations. Advanced data security technologies such as encryption and access controls are vital for compliance with data protection laws.
Leveraging Data Governance Tools and Technology

Leveraging tools and technologies in data governance is essential for enhancing data management effectiveness and compliance. The implementation of data catalogs enhances the effectiveness of data governance by providing a centralized repository for data assets.
Overall, the integration of advanced tools and processes plays a critical role in optimizing data governance initiatives across organizations.
Metadata Management Solutions
Metadata management is crucial for understanding the context and meaning of data assets within an organization. By providing detailed information about data sources, structures, and relationships, metadata management significantly boosts data discoverability.
Governance practices are enhanced through metadata by providing data stewards and owners with crucial insights into data usage and lineage.
Data Catalogs for Effective Data Management
Data catalogs provide a centralized metadata repository for data assets, thus supporting effective governance. Modern data catalogs offer features such as search functions, data lineage documentation, and collaboration tools to streamline data management and enhance user experience.
Effective data discovery through catalogs facilitates better access for data analytics and promotes collaboration, aiding in data democratization.
Advanced Data Security Measures
Information lifecycle and content management technologies are crucial for controlling data volumes and managing risk. Advanced data security measures, including encryption and access controls, are necessary to protect sensitive data and comply with data privacy regulations. Implementing robust security protocols ensures that data remains protected throughout its lifecycle.
Measuring and Monitoring Data Governance Performance

Establishing performance metrics allows organizations to monitor compliance and the quality of data governance initiatives. Identifying the effectiveness of governance programs and ensuring alignment with organizational objectives requires measuring data governance performance.
Utilizing metrics such as compliance audit outcomes and data quality scores can provide valuable insights into governance performance.
Establishing Performance Metrics
Clear and relevant metrics gauge the success of data governance initiatives and ensure accountability. Key performance indicators (KPIs) can include data quality scores, data availability rates, and compliance with data standards.
Measuring baseline metrics before making changes is crucial to demonstrate the effectiveness of data governance efforts over time.
Continuous Improvement and Feedback Loops
Regular assessment of data governance processes informs improvements based on feedback. Feedback loops enable continuous refinement of data governance strategies.
Data governance should be treated as an evolving cycle, integrating continuous feedback to enhance processes over time.
Common Challenges in Data Governance

Data breaches and regulations like GDPR have made privacy protections a central part of governance efforts. Approximately 60% of organizations may not realize the expected business value of AI applications due to governance issues. Significant challenges in data governance include organizational issues, culture shifts, rapid data proliferation, and the need for new procedures.
Overcoming Organizational Resistance
Early and frequent stakeholder engagement boosts the chances of success for data governance initiatives. Cultivating a culture where data literacy is integral to operations empowers all employees to make data-driven decisions.
Managing Rapid Data Proliferation
Rapid growth of data often leads to challenges such as new data being unstructured, which can tax existing systems. Implementing an adaptive data governance approach tailored to individual business processes is recommended to handle the challenges posed by rapid data growth.
Best Practices for Data Governance Initiatives
Defining what data means to an organization is fundamental for effective data governance. Establishing a framework, prioritizing stakeholder buy-in, and ensuring clear communication are critical.
Implementing data governance requires a mindset that combines people, processes, and technology, focusing first on human resources.
Creating a Data Glossary
A data glossary ensures consistent understanding and communication of data definitions. Metadata management solutions enhance data discovery, usage, and governance by maintaining comprehensive definitions and categories.
Data catalogs play a crucial role in effective data management by providing easy access to definitions and fostering collaboration among stakeholders.
Promoting Data Literacy Across the Organization
Business users need to be familiar with data usage rules and privacy mandates to ensure compliance. Data literacy is crucial for employees to effectively use and understand data in their roles.
Training and education programs are essential for empowering staff to make data-driven decisions.
Encouraging Collaboration and Communication
Input from diverse teams enhances data governance programs and fosters a culture of collaboration. Shared goals and collaborative platforms significantly enhance teamwork among data stakeholders.
Establishing cross-functional teams can enhance collaboration, ensuring diverse perspectives are included in data governance efforts.
Case Studies and Examples
Real-world examples demonstrate the practical benefits of implementing data governance frameworks within organizations. A financial institution enhanced compliance and data quality through a robust data governance program, ensuring better management of sensitive financial data.
A healthcare provider improved patient data management and met regulatory compliance requirements by adopting an effective data governance strategy.
Financial Industry Case Study
A prominent financial institution utilized a data governance program to enhance its compliance with regulatory standards, resulting in a marked reduction in data-related penalties. Wells Fargo developed a unified data source to enhance accuracy and streamline reporting, which is crucial for effective governance.
The project involved creating a vendor data registry that improved accountability and provided better insights into data usage across various teams.
Healthcare Sector Example
A healthcare provider enhanced patient data management by implementing a robust data governance framework that prioritized regulatory compliance. The comprehensive data governance practices allowed the healthcare provider to meet HIPAA requirements more effectively.
Utilizing these practices improved the accuracy and accessibility of patient information, ultimately enhancing patient care.
Summary
In summary, mastering the data governance framework involves understanding its importance, building a robust strategy, implementing effective processes, leveraging the right tools, and continuously measuring performance. Overcoming common challenges and adopting best practices can significantly enhance data quality, compliance, and overall data management.
The journey to effective data governance is ongoing, requiring commitment, collaboration, and continuous improvement. By following the guidelines and insights provided in this blog post, organizations can unlock the full potential of their data assets, driving better decision-making and achieving strategic goals.
Frequently Asked Questions
What is a framework in data governance?
A data governance framework is a structured model that establishes guidelines, protocols, and processes for managing enterprise data effectively. It provides a unified approach to ensure proper data collection, storage, and usage within an organization.
Why is data governance important?
Data governance is important as it ensures data integrity, regulatory compliance, and effective usage, which are vital for informed decision-making and risk management. These elements collectively foster a reliable data environment that supports organizational success.
Who is responsible for data governance in an organization?
Data governance in an organization is primarily the responsibility of the Chief Data Officer (CDO) or a dedicated governance manager, along with support from data stewards and the governance team. This structured approach ensures effective oversight and management of data resources.
How can organizations ensure data quality and integrity?
Organizations can ensure data quality and integrity by implementing data quality scores, conducting regular audits, and utilizing data catalogs to track data lineage and usage. This approach fosters a reliable and trustworthy data environment.
What are some common challenges in data governance?
Common challenges in data governance include organizational resistance, difficulties in managing rapid data proliferation, and securing adequate resources for governance initiatives. Addressing these issues is crucial for effective data management.
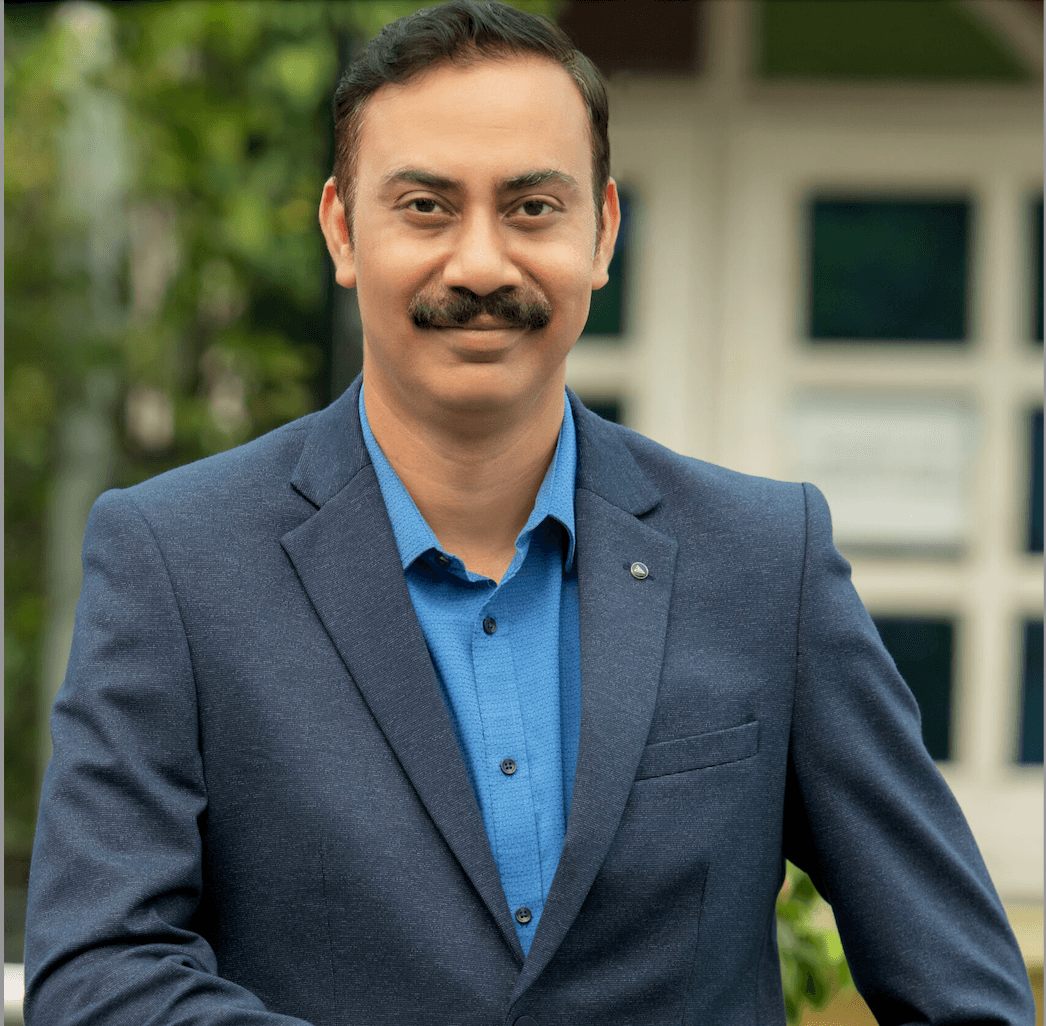
About Pronam Chatterjee
A visionary with 25 years of technical leadership under his belt, Pronam isn’t just ahead of the curve; he’s redefining it. His expertise extends beyond the technical, making him a sought-after speaker and published thought leader.
Whether strategizing the next technology and data innovation or his next chess move, Pronam thrives on pushing boundaries. He is a father of two loving daughters and a Golden Retriever.
With a blend of brilliance, vision, and genuine connection, Pronam is more than a leader; he’s an architect of the future, building something extraordinary
Related Posts
Effective data quality management is crucial for organizations to reduce the costs associated with identifying and fixing bad data.
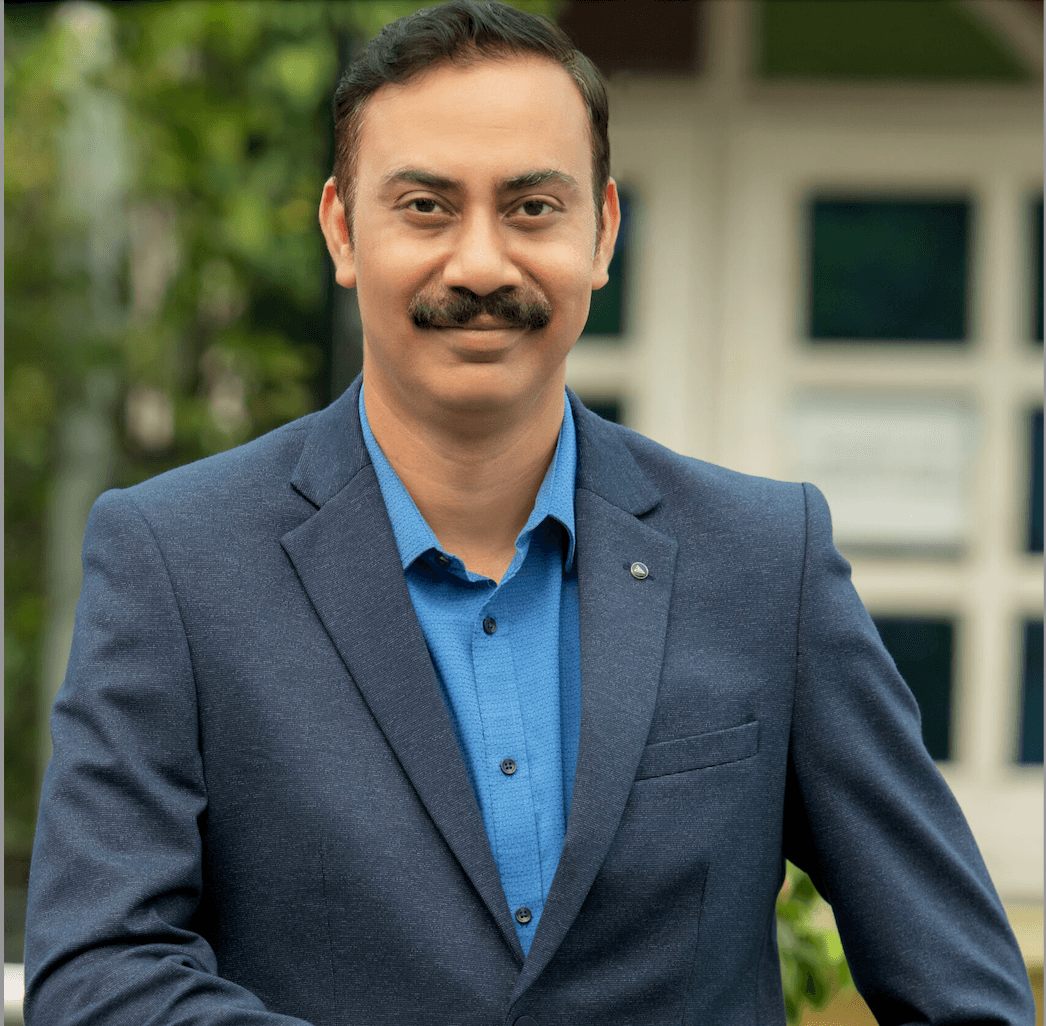
Data privacy means safeguarding personal information from misuse. It’s essential to prevent identity theft and build trust online.
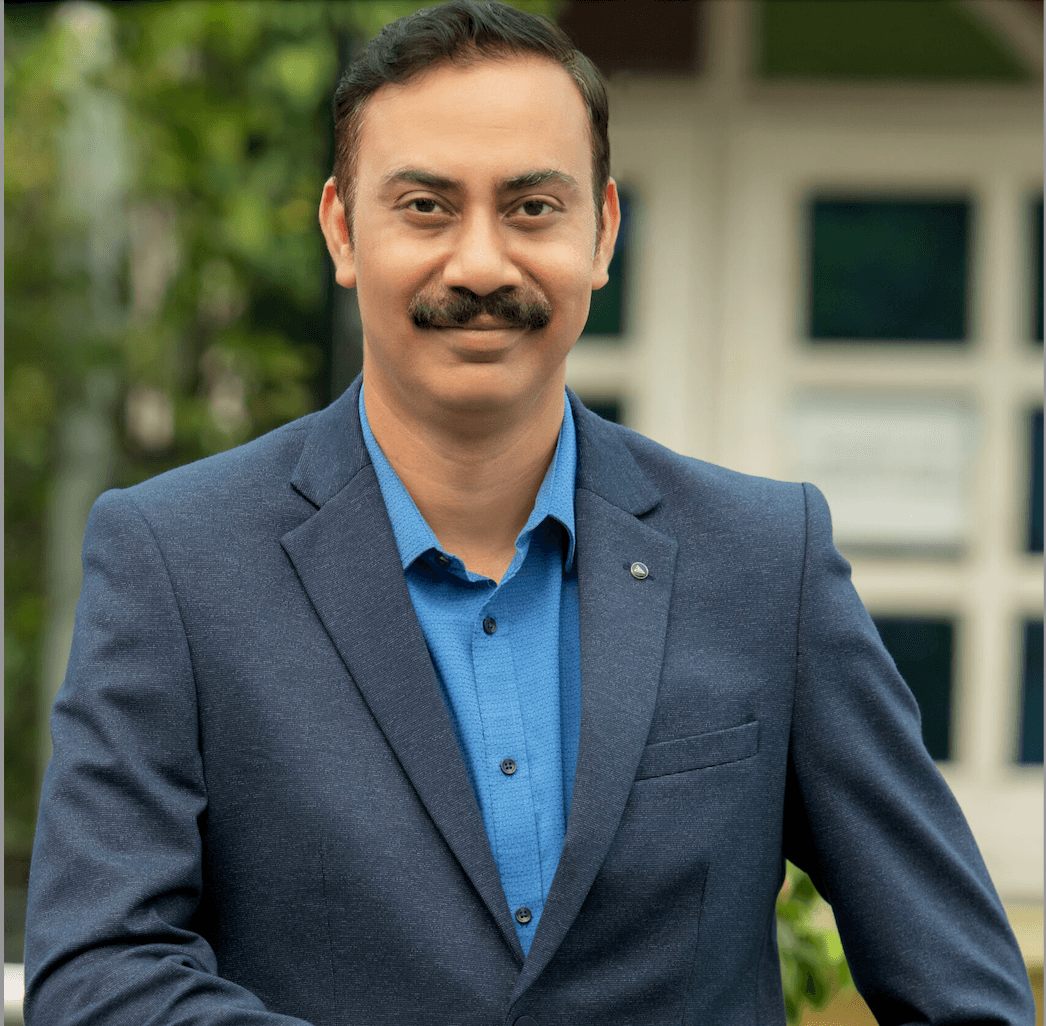
Data engineering involves designing systems to collect, store, and analyze data efficiently.
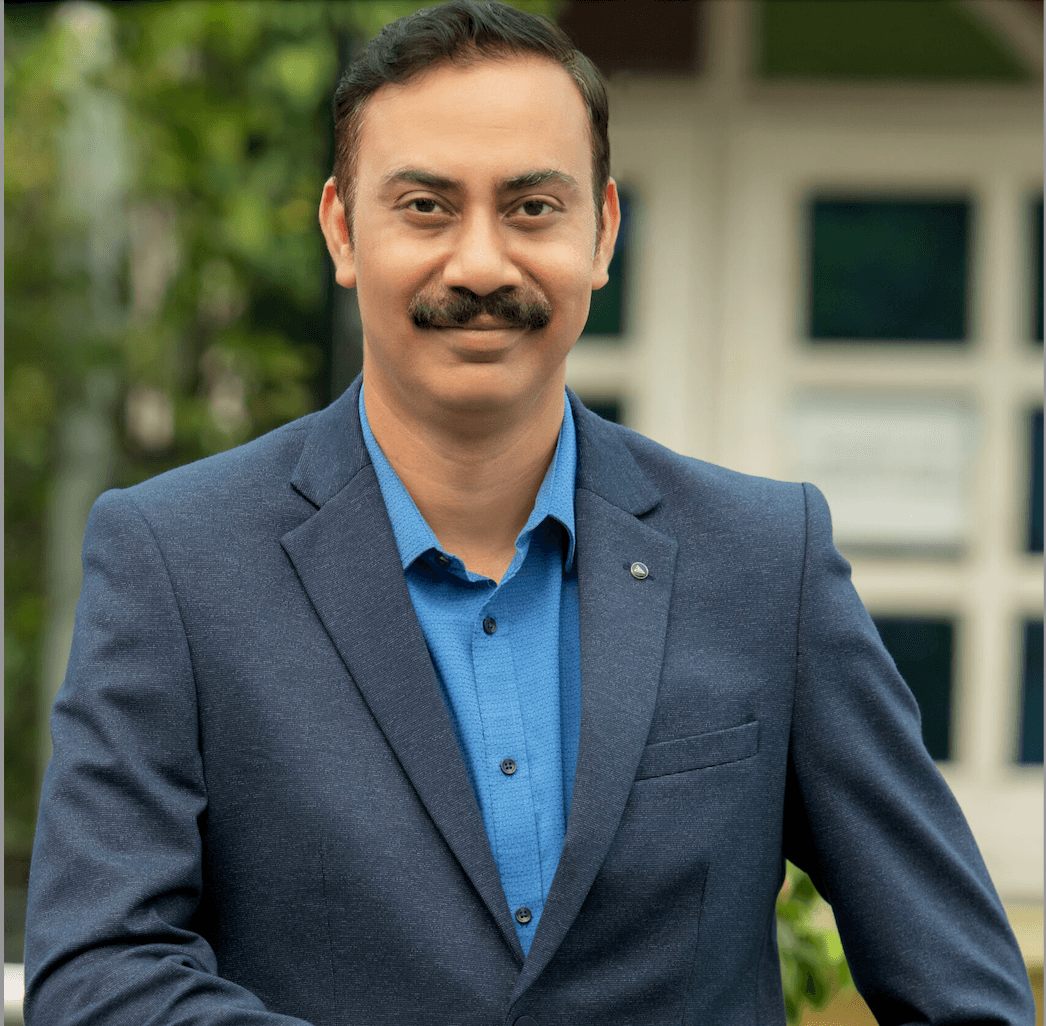